Databricks Certified Associate Developer for Apache Spark Exam Practice Questions (P. 3)
- Full Access (207 questions)
- Six months of Premium Access
- Access to one million comments
- Seamless ChatGPT Integration
- Ability to download PDF files
- Anki Flashcard files for revision
- No Captcha & No AdSense
- Advanced Exam Configuration
Question #11
Which of the following describes the difference between cluster and client execution modes?
- AThe cluster execution mode runs the driver on a worker node within a cluster, while the client execution mode runs the driver on the client machine (also known as a gateway machine or edge node).
- BThe cluster execution mode is run on a local cluster, while the client execution mode is run in the cloud.
- CThe cluster execution mode distributes executors across worker nodes in a cluster, while the client execution mode runs a Spark job entirely on one client machine.
- DThe cluster execution mode runs the driver on the cluster machine (also known as a gateway machine or edge node), while the client execution mode runs the driver on a worker node within a cluster.
- EThe cluster execution mode distributes executors across worker nodes in a cluster, while the client execution mode submits a Spark job from a remote machine to be run on a remote, unconfigurable cluster.
Correct Answer:
A
A
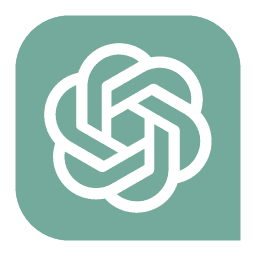
Absolutely, let's hash this out. The key differentiator between cluster and client execution modes in Apache Spark lies where the driver is hosted. In cluster mode, the driver runs on a worker node inside the cluster. This setup can help optimize performance as the driver is closer to the executors. On the other hand, in client mode, the driver operates from the client machine, often referred to as the gateway machine or edge node. This distinction is crucial for understanding how Spark manages resources and jobs across different environments. Essentially, where the driver is located can significantly influence data processing workflows and performance.
send
light_mode
delete
Question #12
Which of the following statements about Spark’s stability is incorrect?
- ASpark is designed to support the loss of any set of worker nodes.
- BSpark will rerun any failed tasks due to failed worker nodes.
- CSpark will recompute data cached on failed worker nodes.
- DSpark will spill data to disk if it does not fit in memory.
- ESpark will reassign the driver to a worker node if the driver’s node fails.Most Voted
Correct Answer:
C
C
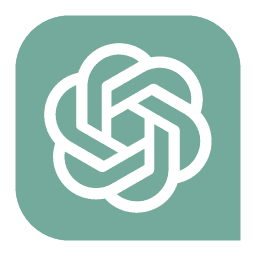
The statement in option E, stating that "Spark will reassign the driver to a worker node if the driver’s node fails," is indeed incorrect. In Apache Spark, the driver plays a crucial role in overseeing the application's execution and is not automatically transferable to another node if its hosting node fails. Instead, the failure of the driver node generally results in the failure of the entire Spark application, necessitating a restart. Spark's architecture does not support the dynamic reassignment of the driver to a worker node as described in this option. This highlights a fundamental aspect of Spark’s management of application execution and fault tolerance.
send
light_mode
delete
Question #13
Which of the following cluster configurations is most likely to experience an out-of-memory error in response to data skew in a single partition?
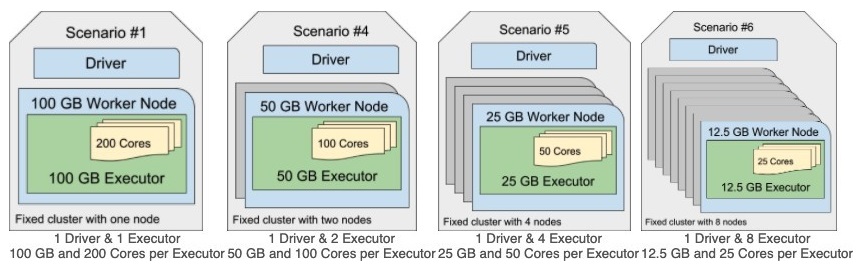
Note: each configuration has roughly the same compute power using 100 GB of RAM and 200 cores.
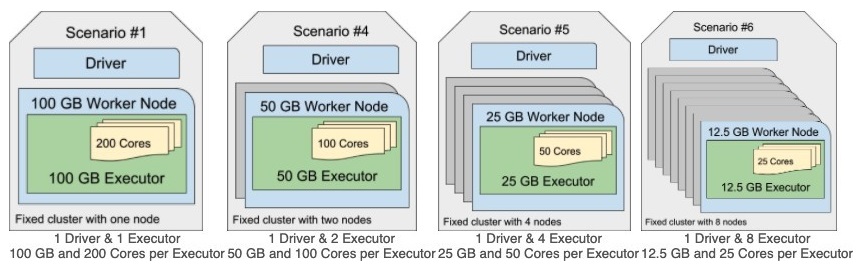
Note: each configuration has roughly the same compute power using 100 GB of RAM and 200 cores.
- AScenario #4
- BScenario #5
- CScenario #6Most Voted
- DMore information is needed to determine an answer.
- EScenario #1
Correct Answer:
C
C
send
light_mode
delete
Question #14
Of the following situations, in which will it be most advantageous to store DataFrame df at the MEMORY_AND_DISK storage level rather than the MEMORY_ONLY storage level?
- AWhen all of the computed data in DataFrame df can fit into memory.
- BWhen the memory is full and it’s faster to recompute all the data in DataFrame df rather than read it from disk.
- CWhen it’s faster to recompute all the data in DataFrame df that cannot fit into memory based on its logical plan rather than read it from disk.
- DWhen it’s faster to read all the computed data in DataFrame df that cannot fit into memory from disk rather than recompute it based on its logical plan.Most Voted
- EThe storage level MENORY_ONLY will always be more advantageous because it’s faster to read data from memory than it is to read data from disk.
Correct Answer:
B
B
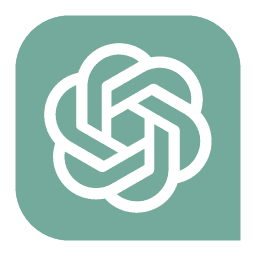
It's crucial to grasp the distinctions in data handling between MEMORY_ONLY and MEMORY_AND_DISK storage levels to answer this query effectively. MEMORY_ONLY omits storing the data that exceeds memory capacity, opting to recompute it when needed. On the other hand, MEMORY_AND_DISK stores overflow data on disk, allowing it to be read from disk rather than recomputed, which can be advantageous when recomputation is more resource-intensive than disk reads. This often results in enhanced performance in scenarios where memory capacity is exceeded, making MEMORY_AND_DISK a more optimal choice under such conditions. Keep in mind, the real-world efficiency gain depends greatly on the specifics of your data and processing needs.
send
light_mode
delete
Question #15
A Spark application has a 128 GB DataFrame A and a 1 GB DataFrame B. If a broadcast join were to be performed on these two DataFrames, which of the following describes which DataFrame should be broadcasted and why?
- AEither DataFrame can be broadcasted. Their results will be identical in result and efficiency.
- BDataFrame B should be broadcasted because it is smaller and will eliminate the need for the shuffling of itself.
- CDataFrame A should be broadcasted because it is larger and will eliminate the need for the shuffling of DataFrame B.
- DDataFrame B should be broadcasted because it is smaller and will eliminate the need for the shuffling of DataFrame A.Most Voted
- EDataFrame A should be broadcasted because it is smaller and will eliminate the need for the shuffling of itself.
Correct Answer:
D
D
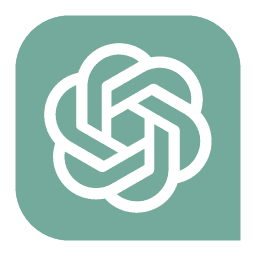
When using a broadcast join in Spark, always opt to broadcast the smaller DataFrame to optimize performance. In this scenario, broadcasting the 1 GB DataFrame B is beneficial because it significantly reduces the requirement to shuffle the large 128 GB DataFrame A across nodes. Broadcasting the smaller DataFrame ensures it is maintained in-memory on each node, simplifying the join process with the larger DataFrame, which is distributed across nodes. This setup prevents the extensive shuffling that would occur if DataFrame A were broadcasted, leading to more efficient joins.
send
light_mode
delete
All Pages