Databricks Certified Associate Developer for Apache Spark Exam Practice Questions (P. 2)
- Full Access (207 questions)
- Six months of Premium Access
- Access to one million comments
- Seamless ChatGPT Integration
- Ability to download PDF files
- Anki Flashcard files for revision
- No Captcha & No AdSense
- Advanced Exam Configuration
Question #6
Which of the following operations is most likely to result in a shuffle?
- ADataFrame.join()Most Voted
- BDataFrame.filter()
- CDataFrame.union()
- DDataFrame.where()
- EDataFrame.drop()
Correct Answer:
A
A
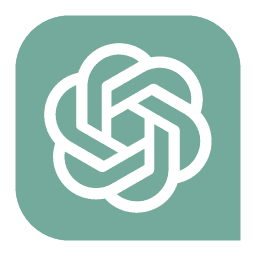
In Apache Spark, the DataFrame.join() operation often leads to shuffling. This is because joining requires the reorganization of data across different partitions to align rows with the same keys in the same executor. This redistribution is necessary to perform the join but can be resource-intensive, causing considerable network and computational overhead. This contrasts with operations like filter or drop, which typically work with data in the current partition and do not require such intensive data movement.
send
light_mode
delete
Question #7
The default value of spark.sql.shuffle.partitions is 200. Which of the following describes what that means?
- ABy default, all DataFrames in Spark will be spit to perfectly fill the memory of 200 executors.
- BBy default, new DataFrames created by Spark will be split to perfectly fill the memory of 200 executors.
- CBy default, Spark will only read the first 200 partitions of DataFrames to improve speed.
- DBy default, all DataFrames in Spark, including existing DataFrames, will be split into 200 unique segments for parallelization.
- EBy default, DataFrames will be split into 200 unique partitions when data is being shuffled.Most Voted
Correct Answer:
E
E
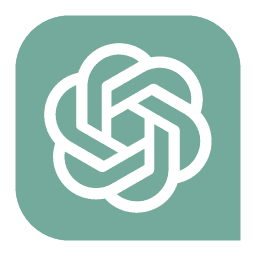
The 'spark.sql.shuffle.partitions' configuration in Apache Spark specifies the standard count of partitions employed during data shuffling, which is pivotal when performing operations like DataFrame joins or aggregations. By default, this value is set to 200, meaning data will get divided into 200 distinct partitions during any shuffle operation. This setup aids in the distribution of tasks across the cluster, allowing for parallel processing and typically improving overall performance. It's crucial, though, to adjust this number based on the specifics of your cluster and dataset to optimize efficiency.
send
light_mode
delete
Question #8
Which of the following is the most complete description of lazy evaluation?
- ANone of these options describe lazy evaluation
- BA process is lazily evaluated if its execution does not start until it is put into action by some type of triggerMost Voted
- CA process is lazily evaluated if its execution does not start until it is forced to display a result to the user
- DA process is lazily evaluated if its execution does not start until it reaches a specified date and time
- EA process is lazily evaluated if its execution does not start until it is finished compiling
Correct Answer:
B
B
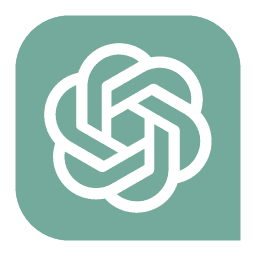
Lazy evaluation is a powerful feature in programming where the execution of certain processes is delayed until absolutely necessary, known as being triggered. This technique not only improves performance by avoiding needless calculations but also maximizes resource utilization by executing only when required. It's extensively leveraged in environments like Apache Spark to optimize data processing tasks, where the execution of a series of transformations waits until an output demands it, enhancing efficiency significantly.
send
light_mode
delete
Question #9
Which of the following DataFrame operations is classified as an action?
- ADataFrame.drop()
- BDataFrame.coalesce()
- CDataFrame.take()Most Voted
- DDataFrame.join()
- EDataFrame.filter()
Correct Answer:
C
C
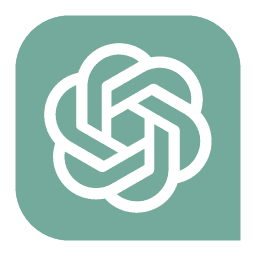
In Spark, the distinction between actions and transformations is crucial. Actions, such as DataFrame.take(), trigger the execution of the logic plan that was built with transformations and give an output. Specifically, DataFrame.take() outputs the first 'n' elements of the DataFrame, executing immediately and providing concrete results, contrasting with transformations which prepare but do not execute data processing workflows. Be sure you understand this for the exam, as recognizing actions is fundamental in managing Spark data processes efficiently.
send
light_mode
delete
Question #10
Which of the following DataFrame operations is classified as a wide transformation?
- ADataFrame.filter()
- BDataFrame.join()Most Voted
- CDataFrame.select()
- DDataFrame.drop()
- EDataFrame.union()
Correct Answer:
B
B
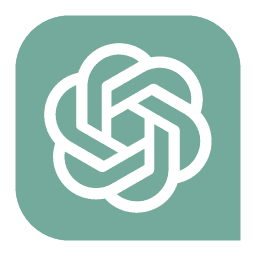
Absolutely right, option B, DataFrame.join(), is indeed a wide transformation. This is because it requires shuffling data across different partitions to combine rows based on a common key. Unlike narrow transformations, which only affect data within a single partition, wide transformations like joins necessitate data movement and redistribution across the network, often leading to more complex execution planning and potential performance impacts.
send
light_mode
delete
All Pages