Snowflake SnowPro Advanced Data Engineer Exam Practice Questions (P. 1)
- Full Access (65 questions)
- Six months of Premium Access
- Access to one million comments
- Seamless ChatGPT Integration
- Ability to download PDF files
- Anki Flashcard files for revision
- No Captcha & No AdSense
- Advanced Exam Configuration
Question #1
A Data Engineer is investigating a query that is taking a long time to return. The Query Profile shows the following:
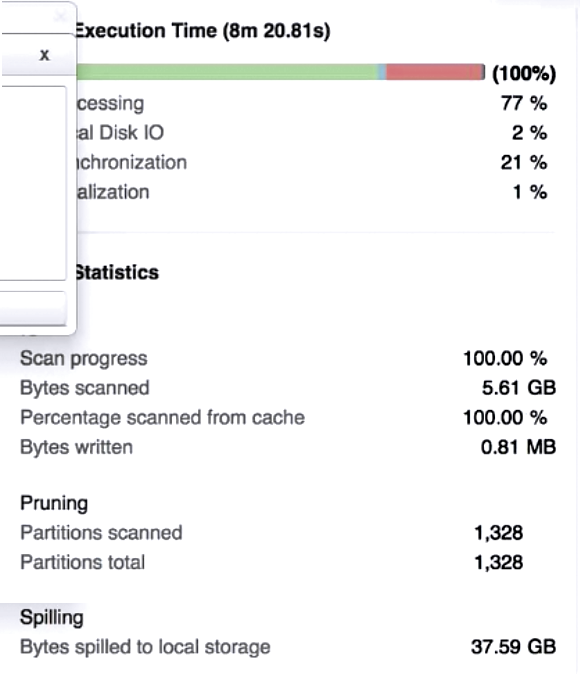
What step should the Engineer take to increase the query performance?
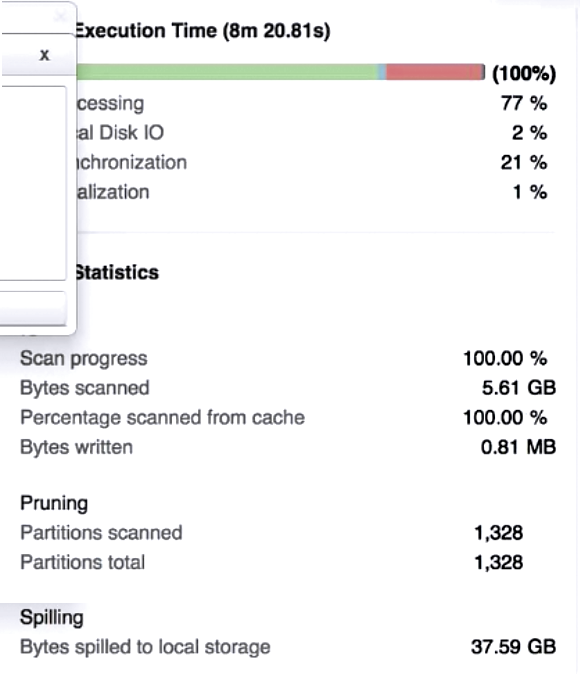
What step should the Engineer take to increase the query performance?
- AAdd additional virtual warehouses.
- BIncrease the size of the virtual warehouse.Most Voted
- CRewrite the query using Common Table Expressions (CTEs).
- DChange the order of the joins and start with smaller tables first.
Correct Answer:
B
B
send
light_mode
delete
Question #2
How can the following relational data be transformed into semi-structured data using the LEAST amount of operational overhead?
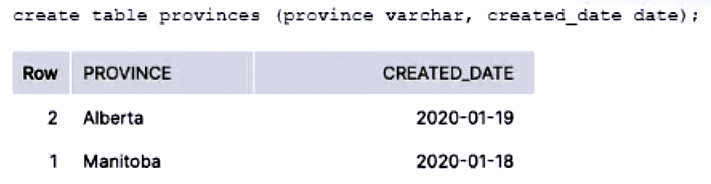
- AUse the TO_JSON function.
- BUse the PARSE_JSON function to produce a VARIANT value.
- CUse the OBJECT_CONSTRUCT function to return a Snowflake object.Most Voted
- DUse the TO_VARIANT function to convert each of the relational columns to VARIANT.
Correct Answer:
C
C
send
light_mode
delete
Question #3
A Data Engineer executes a complex query and wants to make use of Snowflake’s query results caching capabilities to reuse the results.
Which conditions must be met? (Choose three.)
Which conditions must be met? (Choose three.)
- AThe results must be reused within 72 hours.
- BThe query must be executed using the same virtual warehouse.
- CThe USED_CACHED_RESULT parameter must be included in the query.
- DThe table structure contributing to the query result cannot have changed.Most Voted
- EThe new query must have the same syntax as the previously executed query.Most Voted
- FThe micro-partitions cannot have changed due to changes to other data in the table.Most Voted
Correct Answer:
EDF
EDF
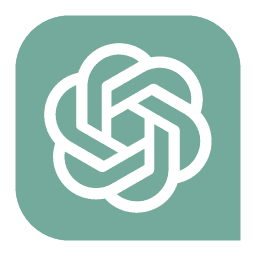
Snowflake's query results caching enables efficiencies by reusing previous query outcomes, but only under specific conditions to ensure data integrity and relevance. Importantly, the original query's structure and the micro-partitions involved must remain unaltered; any changes in these aspects invalidate the cached results. Moreover, the exact syntactic repetition of the query is crucial for the cache to recognize and deliver the previously computed results. This approach helps maintain both speed and consistency in data handling across various queries and sessions without confinement to a single virtual warehouse.
send
light_mode
delete
Question #4
A Data Engineer needs to load JSON output from some software into Snowflake using Snowpipe.
Which recommendations apply to this scenario? (Choose three.)
Which recommendations apply to this scenario? (Choose three.)
- ALoad large files (1 GB or larger).
- BEnsure that data files are 100-250 MB (or larger) in size, compressed.Most Voted
- CLoad a single huge array containing multiple records into a single table row.
- DVerify each value of each unique element stores a single native data type (string or number).Most Voted
- EExtract semi-structured data elements containing null values into relational columns before loading.Most Voted
- FCreate data files that are less than 100 MB and stage them in cloud storage at a sequence greater than once each minute.
Correct Answer:
BDE
BDE
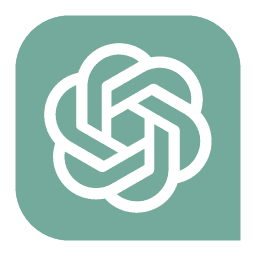
For optimal performance and cost-efficiency when using Snowpipe to load JSON into Snowflake, it's recommended to manage file sizes appropriately. Files should ideally be between 100-250 MB in size, compressed. This helps minimize overhead costs per file, making the process more cost-effective. Moreover, ensuring that each unique data element within the file carries a consistent data type is crucial for maintaining data integrity. Also, handle null values effectively by extracting them into relational columns beforehand, which helps in preserving the structure and quality of your data.
send
light_mode
delete
Question #5
Given the table SALES which has a clustering key of column CLOSED_DATE, which table function will return the average clustering depth for the SALES_REPRESENTATIVE column for the North American region?
- Aselect system$clustering_information('Sales', 'sales_representative', 'region = ''North America''');
- Bselect system$clustering_depth('Sales', 'sales_representative', 'region = ''North America''');Most Voted
- Cselect system$clustering_depth('Sales', 'sales_representative') where region = 'North America';
- Dselect system$clustering_information('Sales', 'sales_representative') where region = 'North America’;
Correct Answer:
B
B
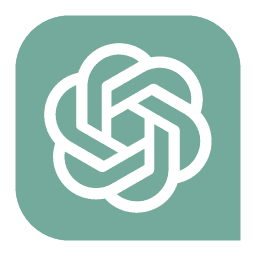
When analyzing the clustering in Snowflake for a specific column within a table that has a condition, the function system$clustering_depth proves to be an efficient choice. It directly returns the average clustering depth linked to the specified column, here 'sales_representative', tailored to a specific filtering condition, 'region = ''North America'''. This function is designed to center its output strictly on the depth aspect, avoiding unnecessary details about other clustering statistics, making it both straightforward and focused for performance evaluation tasks.
send
light_mode
delete
All Pages