Google Professional Machine Learning Engineer Exam Practice Questions (P. 2)
- Full Access (304 questions)
- Six months of Premium Access
- Access to one million comments
- Seamless ChatGPT Integration
- Ability to download PDF files
- Anki Flashcard files for revision
- No Captcha & No AdSense
- Advanced Exam Configuration
Question #11
You are designing an ML recommendation model for shoppers on your company's ecommerce website. You will use Recommendations AI to build, test, and deploy your system. How should you develop recommendations that increase revenue while following best practices?
- AUse the ג€Other Products You May Likeג€ recommendation type to increase the click-through rate.
- BUse the ג€Frequently Bought Togetherג€ recommendation type to increase the shopping cart size for each order.Most Voted
- CImport your user events and then your product catalog to make sure you have the highest quality event stream.
- DBecause it will take time to collect and record product data, use placeholder values for the product catalog to test the viability of the model.
Correct Answer:
B
Frequently bought together' recommendations aim to up-sell and cross-sell customers by providing product.
Reference:
https://rejoiner.com/resources/amazon-recommendations-secret-selling-online/
B
Frequently bought together' recommendations aim to up-sell and cross-sell customers by providing product.
Reference:
https://rejoiner.com/resources/amazon-recommendations-secret-selling-online/
send
light_mode
delete
Question #12
You are designing an architecture with a serverless ML system to enrich customer support tickets with informative metadata before they are routed to a support agent. You need a set of models to predict ticket priority, predict ticket resolution time, and perform sentiment analysis to help agents make strategic decisions when they process support requests. Tickets are not expected to have any domain-specific terms or jargon.
The proposed architecture has the following flow:
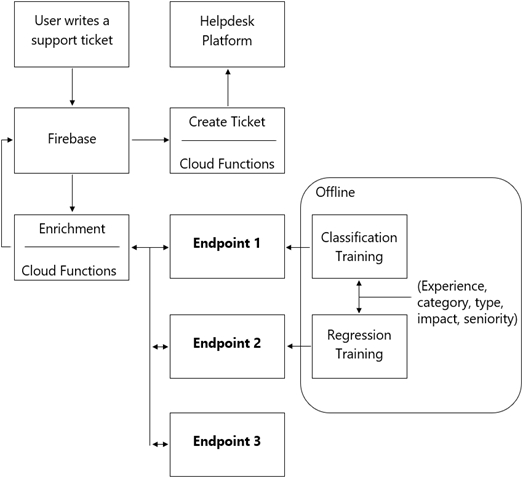
Which endpoints should the Enrichment Cloud Functions call?
The proposed architecture has the following flow:
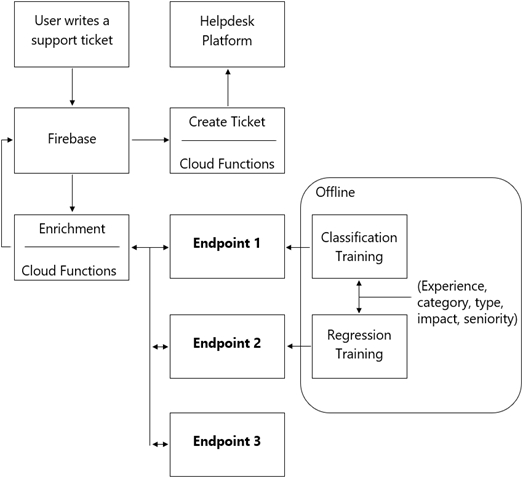
Which endpoints should the Enrichment Cloud Functions call?
- A1 = AI Platform, 2 = AI Platform, 3 = AutoML Vision
- B1 = AI Platform, 2 = AI Platform, 3 = AutoML Natural Language
- C1 = AI Platform, 2 = AI Platform, 3 = Cloud Natural Language APIMost Voted
- D1 = Cloud Natural Language API, 2 = AI Platform, 3 = Cloud Vision API
Correct Answer:
B
B
send
light_mode
delete
Question #13
You have trained a deep neural network model on Google Cloud. The model has low loss on the training data, but is performing worse on the validation data. You want the model to be resilient to overfitting. Which strategy should you use when retraining the model?
- AApply a dropout parameter of 0.2, and decrease the learning rate by a factor of 10.
- BApply a L2 regularization parameter of 0.4, and decrease the learning rate by a factor of 10.
- CRun a hyperparameter tuning job on AI Platform to optimize for the L2 regularization and dropout parameters.Most Voted
- DRun a hyperparameter tuning job on AI Platform to optimize for the learning rate, and increase the number of neurons by a factor of 2.
Correct Answer:
D
D
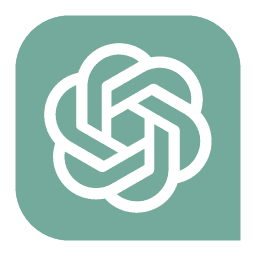
Regarding the choice between using answer D and conducting a hyperparameter tuning job focused on learning rate and increasing neurons, it seems counter-intuitive in terms of minimizing overfitting. Increasing neurons indeed adds complexity, which can exacerbate an overfitting scenario if not properly managed. A more effective approach might indeed involve tuning L2 regularization and dropout, as these methods directly tackle model complexity to enhance generalizability. Opting for a hyperparameter tuning job to ensure that the right balance between model complexity and training dynamics is found generally gives you an advantage in combating overfitting.
send
light_mode
delete
Question #14
You built and manage a production system that is responsible for predicting sales numbers. Model accuracy is crucial, because the production model is required to keep up with market changes. Since being deployed to production, the model hasn't changed; however the accuracy of the model has steadily deteriorated.
What issue is most likely causing the steady decline in model accuracy?
What issue is most likely causing the steady decline in model accuracy?
- APoor data quality
- BLack of model retrainingMost Voted
- CToo few layers in the model for capturing information
- DIncorrect data split ratio during model training, evaluation, validation, and test
Correct Answer:
D
D
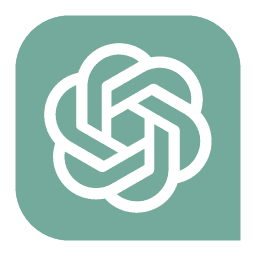
It seems that the notion of "Incorrect data split ratio during model training, evaluation, validation, and test" as the cause of declining model accuracy post-deployment doesn't completely hold up. The essence here lies in the evolving nature of market conditions, which necessitates recurring model updates to maintain accuracy. A model that isn't periodically recalibrated with fresh data will likely fall short as it continues to learn from the initial, now outdated dataset. This suggests that the recurring issue is indeed the lack of retraining, as updating the model to reflect new data trends is crucial in dynamic market environments.
send
light_mode
delete
Question #15
You have been asked to develop an input pipeline for an ML training model that processes images from disparate sources at a low latency. You discover that your input data does not fit in memory. How should you create a dataset following Google-recommended best practices?
- ACreate a tf.data.Dataset.prefetch transformation.
- BConvert the images to tf.Tensor objects, and then run Dataset.from_tensor_slices().
- CConvert the images to tf.Tensor objects, and then run tf.data.Dataset.from_tensors().
- DConvert the images into TFRecords, store the images in Cloud Storage, and then use the tf.data API to read the images for training.Most Voted
Correct Answer:
B
Reference:
https://www.tensorflow.org/api_docs/python/tf/data/Dataset
B
Reference:
https://www.tensorflow.org/api_docs/python/tf/data/Dataset
send
light_mode
delete
Question #16
You are an ML engineer at a large grocery retailer with stores in multiple regions. You have been asked to create an inventory prediction model. Your model's features include region, location, historical demand, and seasonal popularity. You want the algorithm to learn from new inventory data on a daily basis. Which algorithms should you use to build the model?
- AClassification
- BReinforcement Learning
- CRecurrent Neural Networks (RNN)Most Voted
- DConvolutional Neural Networks (CNN)
Correct Answer:
B
Reference:
https://www.kdnuggets.com/2018/03/5-things-reinforcement-learning.html
B
Reference:
https://www.kdnuggets.com/2018/03/5-things-reinforcement-learning.html
send
light_mode
delete
Question #17
You are building a real-time prediction engine that streams files which may contain Personally Identifiable Information (PII) to Google Cloud. You want to use the
Cloud Data Loss Prevention (DLP) API to scan the files. How should you ensure that the PII is not accessible by unauthorized individuals?
Cloud Data Loss Prevention (DLP) API to scan the files. How should you ensure that the PII is not accessible by unauthorized individuals?
- AStream all files to Google Cloud, and then write the data to BigQuery. Periodically conduct a bulk scan of the table using the DLP API.
- BStream all files to Google Cloud, and write batches of the data to BigQuery. While the data is being written to BigQuery, conduct a bulk scan of the data using the DLP API.
- CCreate two buckets of data: Sensitive and Non-sensitive. Write all data to the Non-sensitive bucket. Periodically conduct a bulk scan of that bucket using the DLP API, and move the sensitive data to the Sensitive bucket.
- DCreate three buckets of data: Quarantine, Sensitive, and Non-sensitive. Write all data to the Quarantine bucket. Periodically conduct a bulk scan of that bucket using the DLP API, and move the data to either the Sensitive or Non-Sensitive bucket.Most Voted
Correct Answer:
A
A
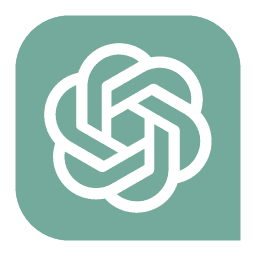
The correct response involves scanning the data for PII using Google Cloud's Data Loss Prevention API before allowing it into your operational data environment. This is efficiently executed by initially writing all streamed files to a single destination like BigQuery, and then performing systematic scans of this destination. An alternative perspective might consider a more continuous or real-time approach as indicated in some user discussions, but such setups could require substantially increased complexity and potential custom development. For standardized implementation on Google Cloud, periodic scanning as described remains a robust choice.
send
light_mode
delete
Question #18
You work for a large hotel chain and have been asked to assist the marketing team in gathering predictions for a targeted marketing strategy. You need to make predictions about user lifetime value (LTV) over the next 20 days so that marketing can be adjusted accordingly. The customer dataset is in BigQuery, and you are preparing the tabular data for training with AutoML Tables. This data has a time signal that is spread across multiple columns. How should you ensure that
AutoML fits the best model to your data?
AutoML fits the best model to your data?
- AManually combine all columns that contain a time signal into an array. AIlow AutoML to interpret this array appropriately. Choose an automatic data split across the training, validation, and testing sets.
- BSubmit the data for training without performing any manual transformations. AIlow AutoML to handle the appropriate transformations. Choose an automatic data split across the training, validation, and testing sets.
- CSubmit the data for training without performing any manual transformations, and indicate an appropriate column as the Time column. AIlow AutoML to split your data based on the time signal provided, and reserve the more recent data for the validation and testing sets.
- DSubmit the data for training without performing any manual transformations. Use the columns that have a time signal to manually split your data. Ensure that the data in your validation set is from 30 days after the data in your training set and that the data in your testing sets from 30 days after your validation set.Most Voted
Correct Answer:
D
D
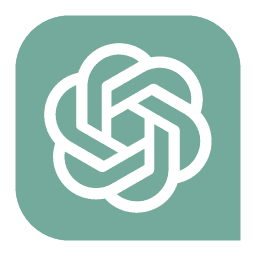
When dealing with a dataset that has time signals spread across multiple columns, it's imperative to manually handle the data splitting for training, validation, and testing. This manual splitting ensures that your model accurately recognizes and utilizes the temporal distribution of the dataset rather than treating each entry with equal historical weight. By designating set periods for training, validation, and testing, the temporal relationships and dependencies within the data are better maintained and reflected in model performance. This approach is crucial for predictive tasks where time plays a significant role in the target variable.
send
light_mode
delete
Question #19
You have written unit tests for a Kubeflow Pipeline that require custom libraries. You want to automate the execution of unit tests with each new push to your development branch in Cloud Source Repositories. What should you do?
- AWrite a script that sequentially performs the push to your development branch and executes the unit tests on Cloud Run.
- BUsing Cloud Build, set an automated trigger to execute the unit tests when changes are pushed to your development branch.Most Voted
- CSet up a Cloud Logging sink to a Pub/Sub topic that captures interactions with Cloud Source Repositories. Configure a Pub/Sub trigger for Cloud Run, and execute the unit tests on Cloud Run.
- DSet up a Cloud Logging sink to a Pub/Sub topic that captures interactions with Cloud Source Repositories. Execute the unit tests using a Cloud Function that is triggered when messages are sent to the Pub/Sub topic.
Correct Answer:
B
B
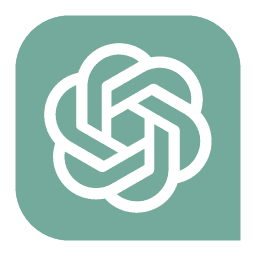
Absolutely, choosing option B is spot on. Cloud Build is designed to handle continuous integration and deployment, which is exactly what you need for automating your unit tests each time there’s a new push in Cloud Source Repositories. It’s straightforward and integrates smoothly with the workflow, unlike other options that add unnecessary complexity. This setup simplifies your CI/CD pipeline by leveraging Cloud Build’s native capabilities, ensuring that your unit tests run automatically and reliably.
send
light_mode
delete
Question #20
You are training an LSTM-based model on AI Platform to summarize text using the following job submission script: gcloud ai-platform jobs submit training $JOB_NAME \
--package-path $TRAINER_PACKAGE_PATH \
--module-name $MAIN_TRAINER_MODULE \
--job-dir $JOB_DIR \
--region $REGION \
--scale-tier basic \
-- \
--epochs 20 \
--batch_size=32 \
--learning_rate=0.001 \
You want to ensure that training time is minimized without significantly compromising the accuracy of your model. What should you do?
--package-path $TRAINER_PACKAGE_PATH \
--module-name $MAIN_TRAINER_MODULE \
--job-dir $JOB_DIR \
--region $REGION \
--scale-tier basic \
-- \
--epochs 20 \
--batch_size=32 \
--learning_rate=0.001 \
You want to ensure that training time is minimized without significantly compromising the accuracy of your model. What should you do?
- AModify the 'epochs' parameter.
- BModify the 'scale-tier' parameter.Most Voted
- CModify the 'batch size' parameter.
- DModify the 'learning rate' parameter.
Correct Answer:
C
C
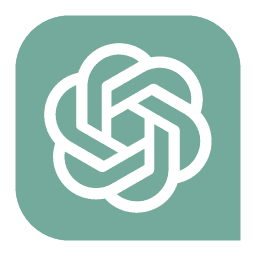
Adjusting the 'batch size' is an effective approach to balancing training time and model accuracy. By increasing the batch size, you can expedite the processing of data through parallelism on capable hardware, potentially reducing training time. However, it's crucial to monitor the model's performance as too large a batch size could adversely affect the accuracy, due to less noise in the gradient estimates. Contrary to some suggestions, changing the scale-tier primarily affects resource allocation rather than directly speeding up training or improving accuracy.
send
light_mode
delete
All Pages