Databricks Certified Machine Learning Professional Exam Practice Questions (P. 3)
- Full Access (60 questions)
- Six months of Premium Access
- Access to one million comments
- Seamless ChatGPT Integration
- Ability to download PDF files
- Anki Flashcard files for revision
- No Captcha & No AdSense
- Advanced Exam Configuration
Question #11
A data scientist is utilizing MLflow to track their machine learning experiments. After completing a series of runs for the experiment with experiment ID exp_id, the data scientist wants to programmatically work with the experiment run data in a Spark DataFrame. They have an active MLflow Client client and an active Spark session spark.
Which of the following lines of code can be used to obtain run-level results for exp_id in a Spark DataFrame?
Which of the following lines of code can be used to obtain run-level results for exp_id in a Spark DataFrame?
- Aclient.list_run_infos(exp_id)
- Bspark.read.format("delta").load(exp_id)
- CThere is no way to programmatically return row-level results from an MLflow Experiment.
- Dmlflow.search_runs(exp_id)
- Espark.read.format("mlflow-experiment").load(exp_id)Most Voted
Correct Answer:
B
B
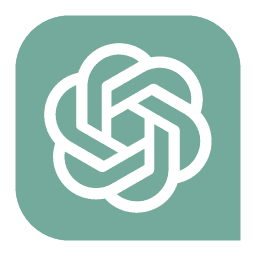
Users highlighted that the correct answer might indeed be E, referring to the Databricks documentation specifically outlining the usage of the format "mlflow-experiment" for loading experiment run data into Spark DataFrame. Based on these references, it seems option E using `spark.read.format("mlflow-experiment").load(exp_id)` can be more suitable for extracting machine learning experiment data directly into a Spark DataFrame concerning format compatibility and specific documentation support.
send
light_mode
delete
Question #12
A data scientist has developed and logged a scikit-learn random forest model model, and then they ended their Spark session and terminated their cluster. After starting a new cluster, they want to review the feature_importances_ of the original model object.
Which of the following lines of code can be used to restore the model object so that feature_importances_ is available?
Which of the following lines of code can be used to restore the model object so that feature_importances_ is available?
- Amlflow.load_model(model_uri)
- Bclient.list_artifacts(run_id)["feature-importances.csv"]
- Cmlflow.sklearn.load_model(model_uri)Most Voted
- DThis can only be viewed in the MLflow Experiments UI
- Eclient.pyfunc.load_model(model_uri)
Correct Answer:
A
A
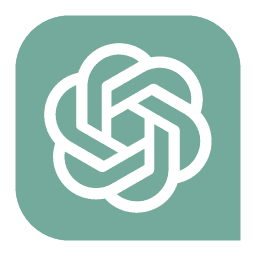
Option C, using mlflow.sklearn.load_model(model_uri), is indeed accurate for loading a scikit-learn model, especially when you need to access specific model attributes like feature_importances_. This method is tailored for scikit-learn, ensuring all the model's properties, including its methods and attributes, are retained and accessible after loading, which is crucial for a thorough analysis or further model evaluation tasks.
send
light_mode
delete
Question #13
Which of the following is a simple statistic to monitor for categorical feature drift?
- AMode
- BNone of these
- CMode, number of unique values, and percentage of missing values
- DPercentage of missing values
- ENumber of unique values
Correct Answer:
C
C
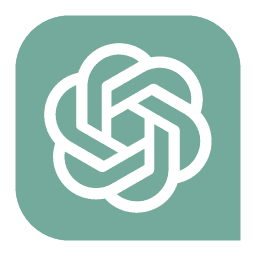
For monitoring categorical feature drift in a dataset, tracking changes in the mode, number of unique values, and percentage of missing values can be very insightful. This combination provides a robust view of any shifts in the distribution or structure of the dataset. The mode helps identify the most frequent category, while tracking the unique values reveals how diverse the categories are. Additionally, monitoring the percentage of missing values is crucial as changes can indicate issues in data collection or processing. Together, these metrics form a comprehensive checkpoint for detecting feature drift in categorical data.
send
light_mode
delete
Question #14
Which of the following is a probable response to identifying drift in a machine learning application?
- ANone of these responses
- BRetraining and deploying a model on more recent dataMost Voted
- CAll of these responses
- DRebuilding the machine learning application with a new label variable
- ESunsetting the machine learning application
Correct Answer:
A
A
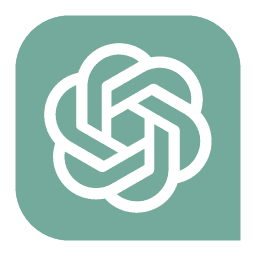
The identification of drift in a machine learning application typically calls for a response that adapts to the new data distribution. Retraining the model with updated or more recent data is a widely accepted practice for preserving model effectiveness. This approach, rather than completely sunsetting the application or rebuilding it with a new label variable, ensures that the model remains relevant and performs well under the evolving conditions.
send
light_mode
delete
Question #15
A data scientist has computed updated feature values for all primary key values stored in the Feature Store table features. In addition, feature values for some new primary key values have also been computed. The updated feature values are stored in the DataFrame features_df. They want to replace all data in features with the newly computed data.
Which of the following code blocks can they use to perform this task using the Feature Store Client fs?
Which of the following code blocks can they use to perform this task using the Feature Store Client fs?
- A
- B
- C
- DMost Voted
- E
Correct Answer:
E
E
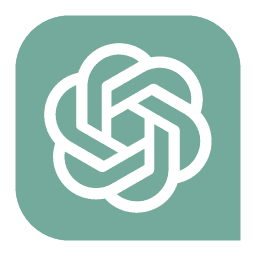
The correct snippet for replacing all data in the features table with newly computed data from features_df must include the use of 'write_table' with the 'overwrite' mode. This is key because 'overwrite' will replace existing entries entirely rather than updating or merging them. The code should essentially invoke 'fs.write_table', specify the table name, reference the DataFrame, and set the mode to 'overwrite', ensuring all previous data is effectively replaced.
send
light_mode
delete
All Pages