Databricks Certified Machine Learning Professional Exam Practice Questions (P. 2)
- Full Access (60 questions)
- Six months of Premium Access
- Access to one million comments
- Seamless ChatGPT Integration
- Ability to download PDF files
- Anki Flashcard files for revision
- No Captcha & No AdSense
- Advanced Exam Configuration
Question #6
A data scientist has developed a model to predict ice cream sales using the expected temperature and expected number of hours of sun in the day. However, the expected temperature is dropping beneath the range of the input variable on which the model was trained.
Which of the following types of drift is present in the above scenario?
Which of the following types of drift is present in the above scenario?
- ALabel drift
- BNone of these
- CConcept drift
- DPrediction drift
- EFeature driftMost Voted
Correct Answer:
E
E
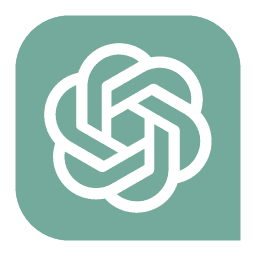
Feature drift is evident when the characteristics of input variables change. Such deviation results in differences in the input feature distribution compared to that at the time of model training. For instance, in this scenario, with temperature values veering below the model's familiar training range, we're confronted with feature drift, as the model now faces values it wasn't originally calibrated to handle. Understanding and identifying this helps maintain model reliability over time.
send
light_mode
delete
Question #7
A data scientist wants to remove the star_rating column from the Delta table at the location path. To do this, they need to load in data and drop the star_rating column.
Which of the following code blocks accomplishes this task?
Which of the following code blocks accomplishes this task?
- Aspark.read.format(“delta”).load(path).drop(“star_rating”)
- Bspark.read.format(“delta”).table(path).drop(“star_rating”)
- CDelta tables cannot be modified
- Dspark.read.table(path).drop(“star_rating”)Most Voted
- Espark.sql(“SELECT * EXCEPT star_rating FROM path”)
Correct Answer:
D
D
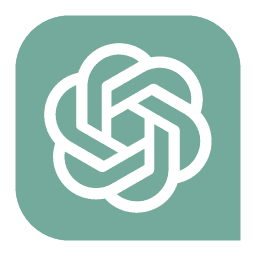
Good catch on option D being incorrect despite being identified as the correct answer. When dealing with file paths, we need to use a method that specifies that we aren't just referring to table names or SQL database tables. In this scenario, where we directly address a Delta table via its file path, option A employs the correct method (`spark.read.format("delta").load(path)`) to load the data. After loading, the `drop("star_rating")` function efficiently removes the designated column. This approach ensures both precision in referencing the data's physical location and functionality in manipulating its structure.
send
light_mode
delete
Question #8
Which of the following operations in Feature Store Client fs can be used to return a Spark DataFrame of a data set associated with a Feature Store table?
- Afs.create_table
- Bfs.write_table
- Cfs.get_table
- DThere is no way to accomplish this task with fs
- Efs.read_tableMost Voted
Correct Answer:
A
A
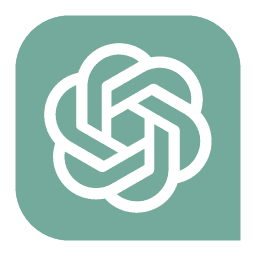
The correct method to retrieve a Spark DataFrame from a Feature Store table is `fs.read_table`. This function specifically serves the purpose of reading data from a feature table, directly returning the data as a Spark DataFrame, which is suitable for further analysis or ML modeling tasks. Therefore, using `fs.read_table` aligns with standard procedures for accessing stored features within a Databricks environment.
send
light_mode
delete
Question #9
A machine learning engineer is in the process of implementing a concept drift monitoring solution. They are planning to use the following steps:
1. Deploy a model to production and compute predicted values
2. Obtain the observed (actual) label values
3. _____
4. Run a statistical test to determine if there are changes over time
Which of the following should be completed as Step #3?
1. Deploy a model to production and compute predicted values
2. Obtain the observed (actual) label values
3. _____
4. Run a statistical test to determine if there are changes over time
Which of the following should be completed as Step #3?
- AObtain the observed values (actual) feature values
- BMeasure the latency of the prediction time
- CRetrain the model
- DNone of these should be completed as Step #3
- ECompute the evaluation metric using the observed and predicted valuesMost Voted
Correct Answer:
D
D
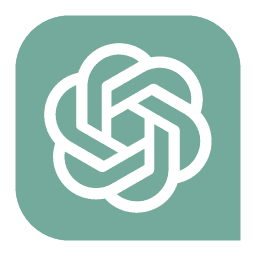
Spot on—the typical drift detection process focuses on identifying changes in the distribution of input data rather than performance metrics. Therefore, none of the given steps (including computing evaluation metrics) fits as Step #3 when solely focusing on statistical tests for identifying this shift over time in concept drift monitoring. Evaluation metrics are key for assessing overall model performance but aren't directly used to detect drift in this particular setup.
send
light_mode
delete
Question #10
Which of the following is a reason for using Jensen-Shannon (JS) distance over a Kolmogorov-Smirnov (KS) test for numeric feature drift detection?
- AAll of these reasons
- BJS is not normalized or smoothed
- CNone of these reasons
- DJS is more robust when working with large datasetsMost Voted
- EJS does not require any manual threshold or cutoff determinations
Correct Answer:
D
D
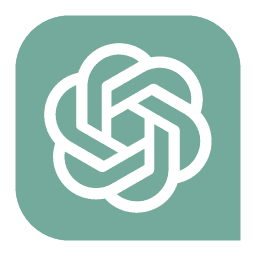
The JS distance offers an advantage when dealing with large datasets because it measures the similarity between probability distributions, making it inherently more robust and scalable. By calculating the divergence between expected and observed distribution data, it effectively helps in identifying drifts, which can be crucial for maintaining the accuracy of models in large-scale applications. This robustness is particularly beneficial in scenarios dealing with complex and vast datasets, where traditional methods might fail or yield less reliable results.
send
light_mode
delete
All Pages