Microsoft AI-900 Exam Practice Questions (P. 5)
- Full Access (246 questions)
- Six months of Premium Access
- Access to one million comments
- Seamless ChatGPT Integration
- Ability to download PDF files
- Anki Flashcard files for revision
- No Captcha & No AdSense
- Advanced Exam Configuration
Question #21
You are building an AI-based app.
You need to ensure that the app uses the principles for responsible AI.
Which two principles should you follow? Each correct answer presents part of the solution.
NOTE: Each correct selection is worth one point.
You need to ensure that the app uses the principles for responsible AI.
Which two principles should you follow? Each correct answer presents part of the solution.
NOTE: Each correct selection is worth one point.
- AImplement an Agile software development methodology
- BImplement a process of AI model validation as part of the software review processMost Voted
- CEstablish a risk governance committee that includes members of the legal team, members of the risk management team, and a privacy officerMost Voted
- DPrevent the disclosure of the use of AI-based algorithms for automated decision making
Correct Answer:
BC
Reference:
https://docs.microsoft.com/en-us/azure/cloud-adoption-framework/innovate/best-practices/trusted-ai https://docs.microsoft.com/en-us/learn/modules/responsible-ai-principles/3-implications-responsible-ai-practical
BC
Reference:
https://docs.microsoft.com/en-us/azure/cloud-adoption-framework/innovate/best-practices/trusted-ai https://docs.microsoft.com/en-us/learn/modules/responsible-ai-principles/3-implications-responsible-ai-practical
send
light_mode
delete
Question #22
HOTSPOT -
To complete the sentence, select the appropriate option in the answer area.
Hot Area:
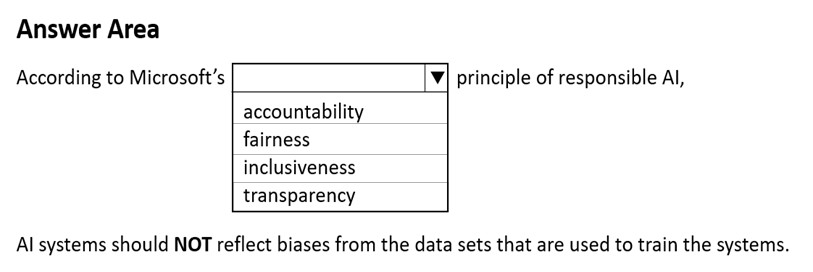
To complete the sentence, select the appropriate option in the answer area.
Hot Area:
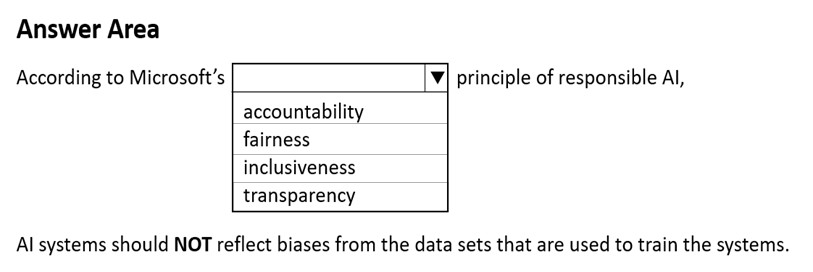
Correct Answer:
Reference:
https://docs.microsoft.com/en-us/azure/cloud-adoption-framework/innovate/best-practices/trusted-ai
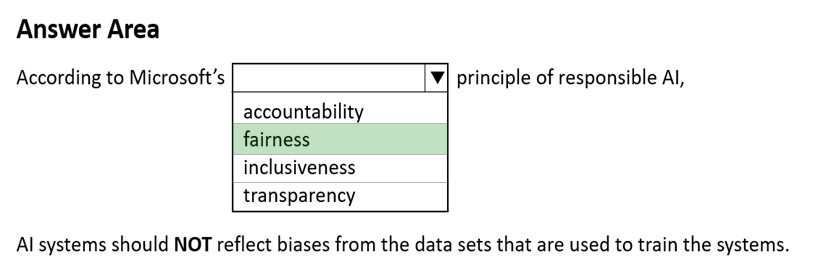
Reference:
https://docs.microsoft.com/en-us/azure/cloud-adoption-framework/innovate/best-practices/trusted-ai
send
light_mode
delete
Question #23
HOTSPOT -
Select the answer that correctly completes the sentence.
Hot Area:
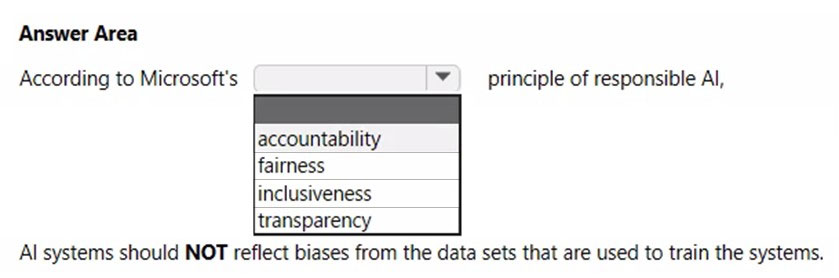
Select the answer that correctly completes the sentence.
Hot Area:
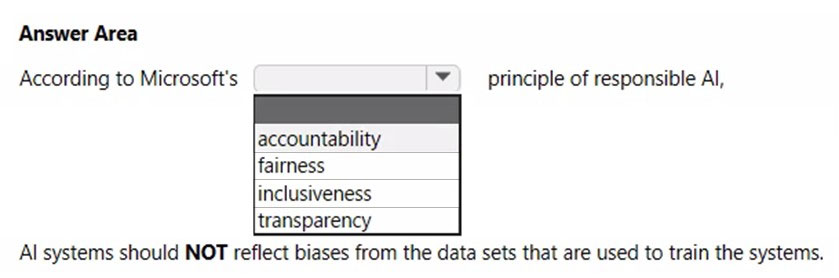
Correct Answer:
Fairness is a core ethical principle that all humans aim to understand and apply. This principle is even more important when AI systems are being developed. Key checks and balances need to make sure that the system's decisions don't discriminate or run a gender, race, sexual orientation, or religion bias toward a group or individual.
Reference:
https://docs.microsoft.com/en-us/azure/cloud-adoption-framework/innovate/best-practices/trusted-ai
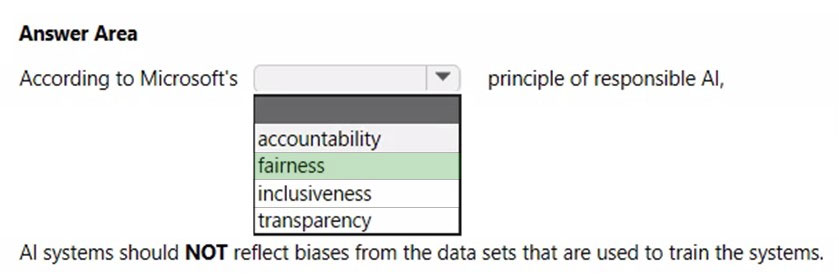
Fairness is a core ethical principle that all humans aim to understand and apply. This principle is even more important when AI systems are being developed. Key checks and balances need to make sure that the system's decisions don't discriminate or run a gender, race, sexual orientation, or religion bias toward a group or individual.
Reference:
https://docs.microsoft.com/en-us/azure/cloud-adoption-framework/innovate/best-practices/trusted-ai
send
light_mode
delete
Question #24
DRAG DROP -
Match the types of AI workloads to the appropriate scenarios.
To answer, drag the appropriate workload type from the column on the left to its scenario on the right. Each workload type may be used once, more than once, or not at all.
NOTE: Each correct selection is worth one point.
Select and Place:
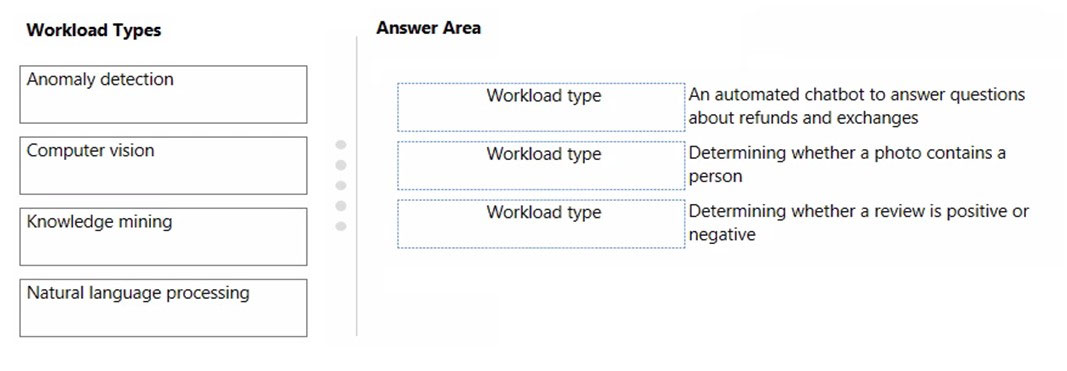
Match the types of AI workloads to the appropriate scenarios.
To answer, drag the appropriate workload type from the column on the left to its scenario on the right. Each workload type may be used once, more than once, or not at all.
NOTE: Each correct selection is worth one point.
Select and Place:
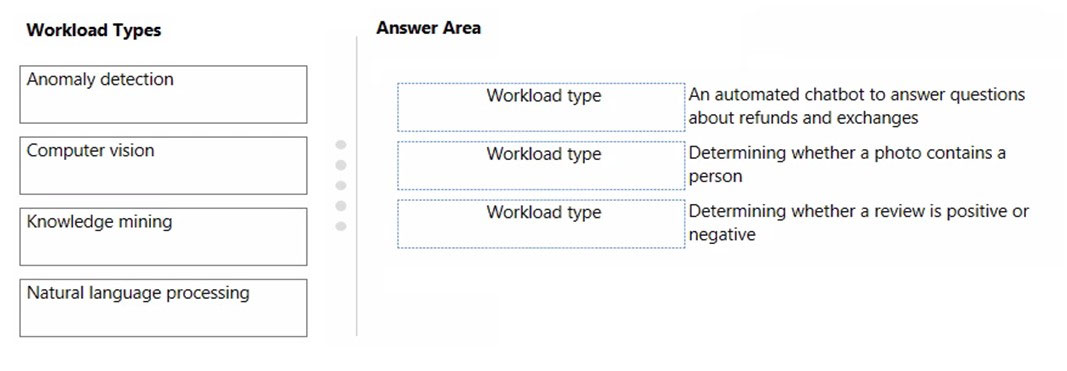
Correct Answer:
Box 1: Knowledge mining -
You can use Azure Cognitive Search's knowledge mining results and populate your knowledge base of your chatbot.
Box 2: Computer vision -
Box 3: Natural language processing
Natural language processing (NLP) is used for tasks such as sentiment analysis.
Reference:
https://docs.microsoft.com/en-us/azure/architecture/data-guide/technology-choices/natural-language-processing
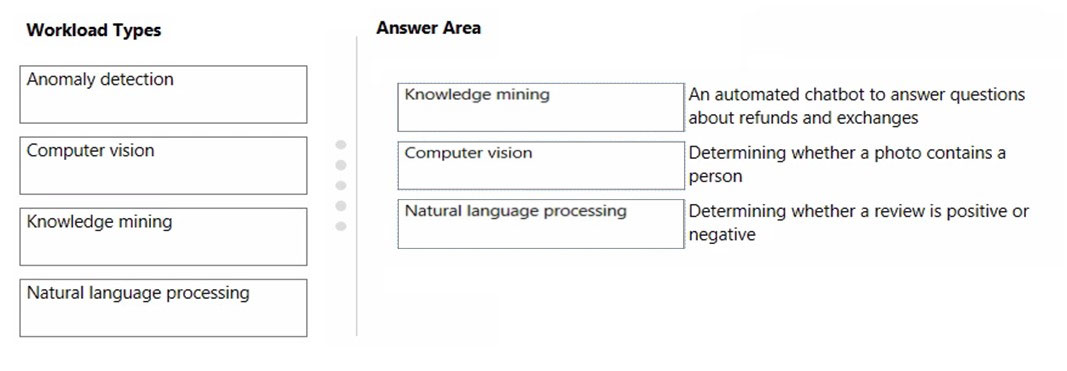
Box 1: Knowledge mining -
You can use Azure Cognitive Search's knowledge mining results and populate your knowledge base of your chatbot.
Box 2: Computer vision -
Box 3: Natural language processing
Natural language processing (NLP) is used for tasks such as sentiment analysis.
Reference:
https://docs.microsoft.com/en-us/azure/architecture/data-guide/technology-choices/natural-language-processing
send
light_mode
delete
Question #25
DRAG DROP -
Match the machine learning tasks to the appropriate scenarios.
To answer, drag the appropriate task from the column on the left to its scenario on the right. Each task may be used once, more than once, or not at all.
NOTE: Each correct selection is worth one point.
Select and Place:
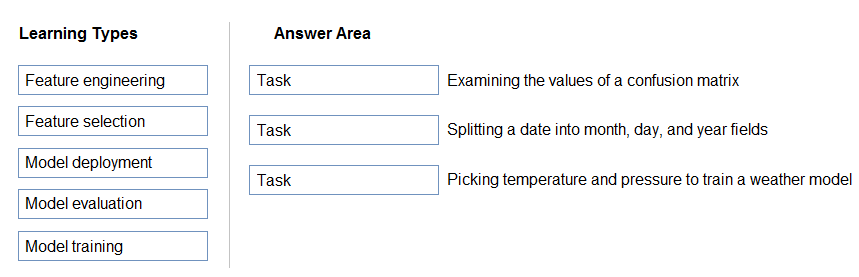
Match the machine learning tasks to the appropriate scenarios.
To answer, drag the appropriate task from the column on the left to its scenario on the right. Each task may be used once, more than once, or not at all.
NOTE: Each correct selection is worth one point.
Select and Place:
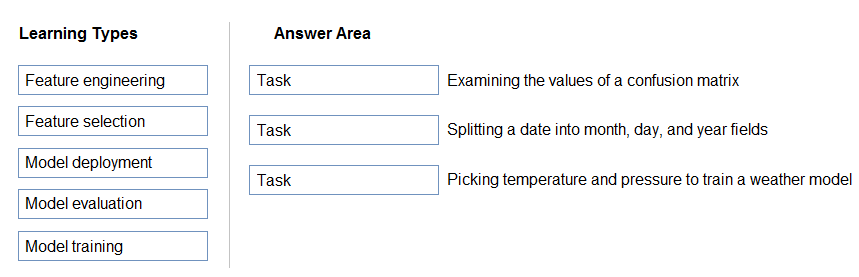
Correct Answer:
Box 1: Model evaluation -
The Model evaluation module outputs a confusion matrix showing the number of true positives, false negatives, false positives, and true negatives, as well as
ROC, Precision/Recall, and Lift curves.
Box 2: Feature engineering -
Feature engineering is the process of using domain knowledge of the data to create features that help ML algorithms learn better. In Azure Machine Learning, scaling and normalization techniques are applied to facilitate feature engineering. Collectively, these techniques and feature engineering are referred to as featurization.
Note: Often, features are created from raw data through a process of feature engineering. For example, a time stamp in itself might not be useful for modeling until the information is transformed into units of days, months, or categories that are relevant to the problem, such as holiday versus working day.
Box 3: Feature selection -
In machine learning and statistics, feature selection is the process of selecting a subset of relevant, useful features to use in building an analytical model. Feature selection helps narrow the field of data to the most valuable inputs. Narrowing the field of data helps reduce noise and improve training performance.
Reference:
https://docs.microsoft.com/en-us/azure/machine-learning/studio/evaluate-model-performance https://docs.microsoft.com/en-us/azure/machine-learning/concept-automated-ml
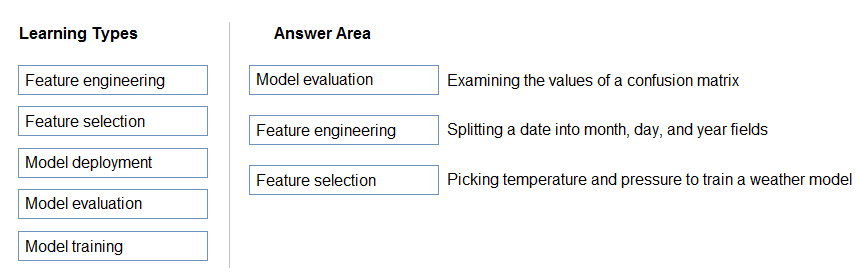
Box 1: Model evaluation -
The Model evaluation module outputs a confusion matrix showing the number of true positives, false negatives, false positives, and true negatives, as well as
ROC, Precision/Recall, and Lift curves.
Box 2: Feature engineering -
Feature engineering is the process of using domain knowledge of the data to create features that help ML algorithms learn better. In Azure Machine Learning, scaling and normalization techniques are applied to facilitate feature engineering. Collectively, these techniques and feature engineering are referred to as featurization.
Note: Often, features are created from raw data through a process of feature engineering. For example, a time stamp in itself might not be useful for modeling until the information is transformed into units of days, months, or categories that are relevant to the problem, such as holiday versus working day.
Box 3: Feature selection -
In machine learning and statistics, feature selection is the process of selecting a subset of relevant, useful features to use in building an analytical model. Feature selection helps narrow the field of data to the most valuable inputs. Narrowing the field of data helps reduce noise and improve training performance.
Reference:
https://docs.microsoft.com/en-us/azure/machine-learning/studio/evaluate-model-performance https://docs.microsoft.com/en-us/azure/machine-learning/concept-automated-ml
send
light_mode
delete
All Pages