Amazon AWS Certified Data Analytics - Specialty Exam Practice Questions (P. 2)
- Full Access (164 questions)
- Six months of Premium Access
- Access to one million comments
- Seamless ChatGPT Integration
- Ability to download PDF files
- Anki Flashcard files for revision
- No Captcha & No AdSense
- Advanced Exam Configuration
Question #6
A company has a business unit uploading .csv files to an Amazon S3 bucket. The company's data platform team has set up an AWS Glue crawler to do discovery, and create tables and schemas. An AWS Glue job writes processed data from the created tables to an Amazon Redshift database. The AWS Glue job handles column mapping and creating the Amazon Redshift table appropriately. When the AWS Glue job is rerun for any reason in a day, duplicate records are introduced into the Amazon Redshift table.
Which solution will update the Redshift table without duplicates when jobs are rerun?
Which solution will update the Redshift table without duplicates when jobs are rerun?
- AModify the AWS Glue job to copy the rows into a staging table. Add SQL commands to replace the existing rows in the main table as postactions in the DynamicFrameWriter class.Most Voted
- BLoad the previously inserted data into a MySQL database in the AWS Glue job. Perform an upsert operation in MySQL, and copy the results to the Amazon Redshift table.
- CUse Apache Spark's DataFrame dropDuplicates() API to eliminate duplicates and then write the data to Amazon Redshift.
- DUse the AWS Glue ResolveChoice built-in transform to select the most recent value of the column.
Correct Answer:
B
B
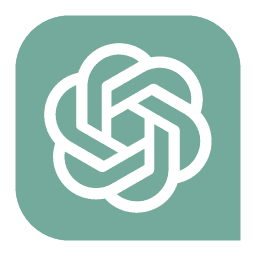
The correct implementation to ensure that duplicates are not introduced when re-running AWS Glue jobs that write to an Amazon Redshift table involves modifying the job to utilize a staging table. You can then manage the integration from staging to production tables through SQL operations that handle data merging or replacement. This solution is efficient because Redshift does not natively support upsert operations directly in a single operation, making the staging table approach necessary for maintaining data integrity. Despite any potential costs or complexity introduced by this setup, it remains a requisite practice to avoid duplication.
send
light_mode
delete
Question #7
A streaming application is reading data from Amazon Kinesis Data Streams and immediately writing the data to an Amazon S3 bucket every 10 seconds. The application is reading data from hundreds of shards. The batch interval cannot be changed due to a separate requirement. The data is being accessed by Amazon
Athena. Users are seeing degradation in query performance as time progresses.
Which action can help improve query performance?
Athena. Users are seeing degradation in query performance as time progresses.
Which action can help improve query performance?
- AMerge the files in Amazon S3 to form larger files.Most Voted
- BIncrease the number of shards in Kinesis Data Streams.
- CAdd more memory and CPU capacity to the streaming application.
- DWrite the files to multiple S3 buckets.
Correct Answer:
C
C
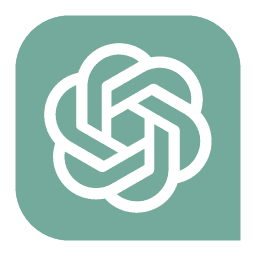
To effectively enhance query performance in Amazon Athena, merging smaller files into larger ones within the Amazon S3 bucket proves to be an efficient strategy. This approach reduces the number of files Athena needs to scan, consequently minimizing the overhead of handling numerous small file metadata and operations. Large files optimize read efficiency by lowering the I/O overhead during query execution. The concerned issue of performance degradation is more directly addressed by optimizing file size rather than enhancing compute resources or modifying the data input flow through additional shards or separate bucket strategies.
send
light_mode
delete
Question #8
A company uses Amazon OpenSearch Service (Amazon Elasticsearch Service) to store and analyze its website clickstream data. The company ingests 1 TB of data daily using Amazon Kinesis Data Firehose and stores one day's worth of data in an Amazon ES cluster.
The company has very slow query performance on the Amazon ES index and occasionally sees errors from Kinesis Data Firehose when attempting to write to the index. The Amazon ES cluster has 10 nodes running a single index and 3 dedicated master nodes. Each data node has 1.5 TB of Amazon EBS storage attached and the cluster is configured with 1,000 shards. Occasionally, JVMMemoryPressure errors are found in the cluster logs.
Which solution will improve the performance of Amazon ES?
The company has very slow query performance on the Amazon ES index and occasionally sees errors from Kinesis Data Firehose when attempting to write to the index. The Amazon ES cluster has 10 nodes running a single index and 3 dedicated master nodes. Each data node has 1.5 TB of Amazon EBS storage attached and the cluster is configured with 1,000 shards. Occasionally, JVMMemoryPressure errors are found in the cluster logs.
Which solution will improve the performance of Amazon ES?
- AIncrease the memory of the Amazon ES master nodes.
- BDecrease the number of Amazon ES data nodes.
- CDecrease the number of Amazon ES shards for the index.Most Voted
- DIncrease the number of Amazon ES shards for the index.
Correct Answer:
C
C
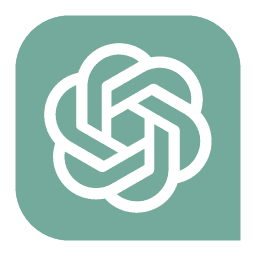
Decreasing the number of shards in your Amazon ES cluster can significantly improve query performance and reduce JVM memory pressure errors. This is particularly true when a cluster is configured with an unusually high number of shards relative to the data volume, as excessive shards can strain node resources unnecessarily. Consolidating your shards will result in fewer, but larger and more manageable index segments, enhancing the overall efficiency of the cluster operations. This adjustment addresses the core issue of resource overutilization and helps balance the load more evenly across cluster nodes.
send
light_mode
delete
Question #9
A manufacturing company has been collecting IoT sensor data from devices on its factory floor for a year and is storing the data in Amazon Redshift for daily analysis. A data analyst has determined that, at an expected ingestion rate of about 2 TB per day, the cluster will be undersized in less than 4 months. A long-term solution is needed. The data analyst has indicated that most queries only reference the most recent 13 months of data, yet there are also quarterly reports that need to query all the data generated from the past 7 years. The chief technology officer (CTO) is concerned about the costs, administrative effort, and performance of a long-term solution.
Which solution should the data analyst use to meet these requirements?
Which solution should the data analyst use to meet these requirements?
- ACreate a daily job in AWS Glue to UNLOAD records older than 13 months to Amazon S3 and delete those records from Amazon Redshift. Create an external table in Amazon Redshift to point to the S3 location. Use Amazon Redshift Spectrum to join to data that is older than 13 months.Most Voted
- BTake a snapshot of the Amazon Redshift cluster. Restore the cluster to a new cluster using dense storage nodes with additional storage capacity.
- CExecute a CREATE TABLE AS SELECT (CTAS) statement to move records that are older than 13 months to quarterly partitioned data in Amazon Redshift Spectrum backed by Amazon S3.
- DUnload all the tables in Amazon Redshift to an Amazon S3 bucket using S3 Intelligent-Tiering. Use AWS Glue to crawl the S3 bucket location to create external tables in an AWS Glue Data Catalog. Create an Amazon EMR cluster using Auto Scaling for any daily analytics needs, and use Amazon Athena for the quarterly reports, with both using the same AWS Glue Data Catalog.
Correct Answer:
B
B
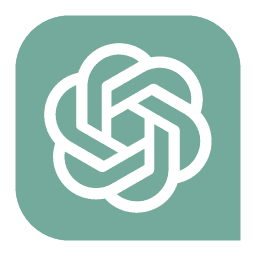
The appropriate solution in this context involves employing AWS Glue to systematically unload data older than 13 months from Redshift to S3, and subsequently directing queries for this older data through Redshift Spectrum. This method is not only efficient for handling large data volumes but also cost-effective, as it leverages S3 for long-term storage while maintaining quick access to recent data via Redshift, effectively addressing the CTO's concerns about costs, administrative efforts, and performance. Option A, which involves AWS Glue and Spectrum, optimally satisfies these requisites by balancing data availability with infrastructure scalability.
send
light_mode
delete
Question #10
An insurance company has raw data in JSON format that is sent without a predefined schedule through an Amazon Kinesis Data Firehose delivery stream to an
Amazon S3 bucket. An AWS Glue crawler is scheduled to run every 8 hours to update the schema in the data catalog of the tables stored in the S3 bucket. Data analysts analyze the data using Apache Spark SQL on Amazon EMR set up with AWS Glue Data Catalog as the metastore. Data analysts say that, occasionally, the data they receive is stale. A data engineer needs to provide access to the most up-to-date data.
Which solution meets these requirements?
Amazon S3 bucket. An AWS Glue crawler is scheduled to run every 8 hours to update the schema in the data catalog of the tables stored in the S3 bucket. Data analysts analyze the data using Apache Spark SQL on Amazon EMR set up with AWS Glue Data Catalog as the metastore. Data analysts say that, occasionally, the data they receive is stale. A data engineer needs to provide access to the most up-to-date data.
Which solution meets these requirements?
- ACreate an external schema based on the AWS Glue Data Catalog on the existing Amazon Redshift cluster to query new data in Amazon S3 with Amazon Redshift Spectrum.
- BUse Amazon CloudWatch Events with the rate (1 hour) expression to execute the AWS Glue crawler every hour.
- CUsing the AWS CLI, modify the execution schedule of the AWS Glue crawler from 8 hours to 1 minute.
- DRun the AWS Glue crawler from an AWS Lambda function triggered by an S3:ObjectCreated:* event notification on the S3 bucket.Most Voted
Correct Answer:
A
A
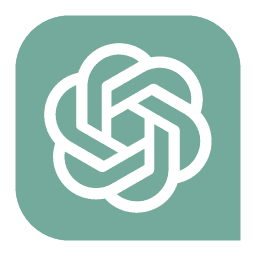
To provide the most up-to-date data availability in this scenario, triggering an AWS Glue Crawler through an AWS Lambda function upon each relevant S3:ObjectCreated event ensures data freshness effectively. This approach would substantially minimize the latency between the data landing in the S3 bucket and its availability for querying via the AWS Glue Data Catalog for analysts using Apache Spark SQL on EMR. This event-driven approach avoids the delay inherent in scheduled crawlers, thereby offering data in near real-time as it arrives.
send
light_mode
delete
All Pages